CV+DL will revolutionize strain screening and biological process monitoring!
In recent years, the combination of computer vision (CV) and deep learning (DL) technologies has brought automated and high-precision solutions to this field.
Enable image assisted intelligent decision-making
Computer vision can accurately analyze the morphology and features of microorganisms through techniques such as image segmentation and feature extraction. Deep learning models, especially convolutional neural networks (CNNs), learn classification and prediction rules from massive amounts of data, providing powerful analytical capabilities for microbial detection.
For example, research has shown that 31 CNN models (such as ResNet and EfficientNet) have been used for colony classification, and transfer learning can quickly adapt to microbial image tasks, greatly reducing training costs.
At the same time, the optimized YOLOv7 model added a small object detection layer and combined it with K-means optimization anchor box calculation, significantly improving the accuracy of colony counting, mAP@.5 An increase of 4.5% indicates that the adjustment of the model structure played a key role in improving performance.
Let computer vision assist in strain screening
The traditional strain screening method relies on microscopic observation and manual counting, which is not only inefficient, but also subjective and prone to errors. Firstly, in terms of high-precision bacterial identification, the classification accuracy of the CNN based DIBaS database (including 660 images of 33 bacteria) is as high as 97.24%.
In addition, a classification model for bacterial colonies such as Escherichia coli and Pseudomonas aeruginosa was successfully constructed using 1252 images of culture dishes captured by a 12 megapixel mobile phone, verifying the feasibility of efficient strain screening using low-cost equipment.
Secondly, in response to the problem of small sample datasets, researchers have improved the model's generalization ability through data augmentation and transfer learning.
For example, in a small dataset of 606 fungal images, these methods perform well in advanced classification units such as phyla and classes.
The top achievements, representative technologies, trillion dollar industries, and future of "big language model+biomanufacturing"!
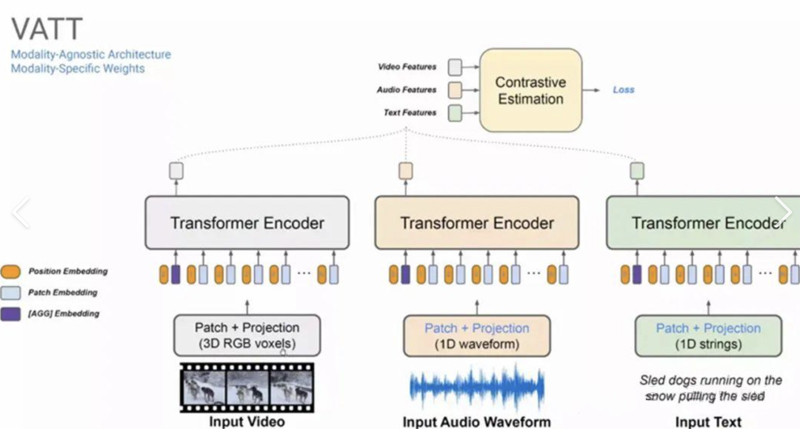
Optimize biological process monitoring through deep learning
In biological processes such as fermentation and pharmaceuticals, the growth dynamics of microorganisms directly affect product quality and production efficiency. CV+DL technology can monitor microbial growth in real-time and provide data support for optimizing production parameters.
On the one hand, through dynamic growth analysis and the combination of conventional cameras and hyperspectral cameras, the time window for image acquisition after plant transformation can be accurately determined, thereby optimizing the production efficiency of bioreactors and ensuring the efficient operation of biological processes.
On the other hand, in terms of pollution source tracking, deep learning analysis of fungal growth images (including 17000 indoor/food fungi) can quickly locate pollution sources and ensure food safety, which is of great significance for quality control of biological processes.